Cognigy AI: Difference Between Lexicon vs. Knowledge
- Dr. Franco Arda
- 21 hours ago
- 3 min read
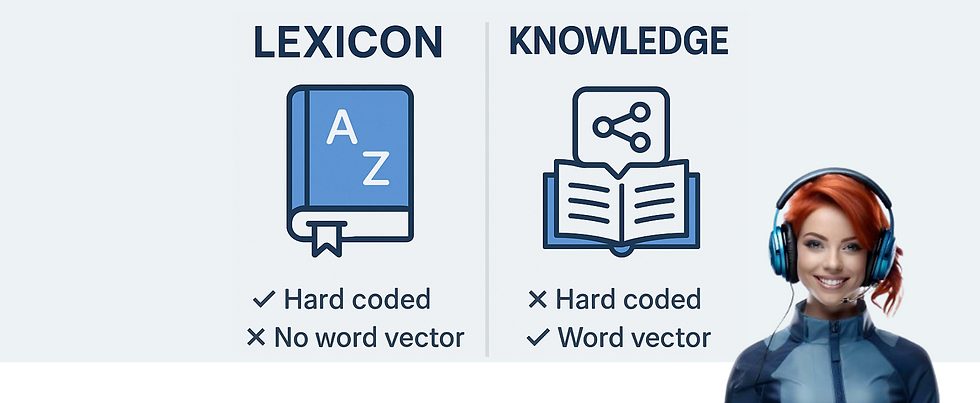
When building conversational AI with Cognigy, one of the keys to delivering a high-performing virtual agent is understanding how it understands language. Cognigy offers two powerful, but distinct, tools to enhance your bot’s comprehension: Lexicon and Knowledge.
Though they may appear similar at first glance — both aim to improve how bots interpret user input — they are fundamentally different in purpose, design, and underlying technology. In this post, we’ll break down the differences and explore when and why you should use each. 🔤 Lexicon: Rule-Based Synonym Mapping
Lexicon in Cognigy is a simple yet powerful feature that lets you define synonym lists or normalization rules. It’s essentially a way to standardize the vocabulary that users might use, mapping different words or phrases to a canonical form before the Natural Language Understanding (NLU) engine attempts to match intents.
For example: {
"New York": ["NYC", "Big Apple", "New York City"]
}
If a user says, “Book a flight to NYC,” Cognigy automatically substitutes “NYC” with “New York” during the intent matching phase — thanks to your Lexicon.
🧠 How It Works:
Static and hardcoded: All mappings are manually defined by the developer or designer.
Pre-processing only: Lexicon entries are applied before intent recognition.
No machine learning involved: No vectorization, no embeddings, just deterministic substitutions.
Perfect for domain-specific language: Use it to normalize product names, acronyms, abbreviations, or regional expressions.
Lexicons shine in scenarios where language is predictable and variant-rich — such as customer service bots, booking systems, or internal help desks. 📚 Knowledge: Semantic Answer Retrieval
Knowledge in Cognigy, on the other hand, is designed for a completely different task: retrieving answers from structured or semi-structured data. Think of it as embedding a lightweight, intelligent FAQ system into your bot — one that can handle real-world phrasing and complexity.
For example:
User asks: “How do I get a refund?”
Bot retrieves: “To request a refund, go to your account settings and select ‘Return & Refunds’.”
🧠 How It Works:
Powered by semantic search: User questions and document content are vectorized using NLP models (like sentence transformers).
Context-aware matching: It understands that “How do I get my money back?” and “What’s your refund policy?” are semantically similar.
Dynamic: You can point it to PDFs, Word docs, or webpages — and it continuously draws from them.
No intent training required: It works out-of-the-box without needing hand-crafted utterances.
This makes Knowledge ideal for scaling answers across complex documentation — such as company policies, product specs, or technical manuals — with minimal effort. 🧠 Lexicon vs. Knowledge — A Smart Comparison
Feature | Lexicon | Knowledge |
🔍 Purpose | Normalize variants for NLU | Answer factual questions using documents |
🛠️ Type | Rule-based, hardcoded | Vector-based, AI-driven |
🧬 NLP Model | None | Embedding models (e.g., SBERT) |
📦 Data Format | Key-value synonym lists | Rich text files, URLs, structured data |
🧭 Use Case | Improve intent matching | Answer unstructured or open-ended questions |
🔗 Dependency | Intent-based logic | Standalone Q&A engine |
💡 When to Use What?
Use Lexicon when:
You want to control vocabulary across intents.
Users may use multiple terms for the same concept (e.g., "mobile", "cellphone", "smartphone").
You’re working with a narrow domain with lots of synonyms or abbreviations.
Use Knowledge when:
Users might ask open-ended, factual questions.
You have lots of documents, FAQs, or policy manuals to draw from.
You want to reduce manual intent creation while still giving accurate answers.
Final Thoughts
Lexicon and Knowledge are not competing features — they’re complementary. Lexicon makes your NLU smarter by ensuring consistency in how inputs are interpreted. Knowledge makes your bot more informative by giving it a memory bank of trusted answers.
Mastering the difference between the two isn’t just a technical win — it’s a design strategy that helps your virtual agent scale with intelligence and elegance.
Comments